Our concern now is to decide how to compare the observed September average extent with the climatological extent, and a prediction. While mine wasn't the best guess in the June summary at ARCUS, it was mine, so I know what the author had in mind.
Let's say that the true number will be 5.25 million km^2. My prediction was 4.92. The useless approach is to look at the two figures, see that they're different, and declare that my prediction was worthless. Now it might be, but you don't know that from just the fact that the prediction and the observation were different. Another part of my prediction was to note that the standard deviation to the prediction was 0.47 million km^2. That is a measure of the 'weather' involved in sea ice extents -- the September average extent has that much variation just because weather happens. Consequently, even if I were absolutely correct -- about the mean (most likely value) and the standard deviation, I'd expect my prediction to be 'wrong' most of the time. 'Wrong' in that useless sense that the observation differed by some observable amount from my prediction. The more useful approach is to allow for the fact that the predicted value really represents a distribution of possibilities -- while 4.92 is the most likely value from my prediction, 5.25 is still quite possible.
We also like to have a 'null forecaster' to compare with. The 'null forecaster' is a particularly simple forecaster, one with no brains to speak of, and very little memory. You always want your prediction to do better than the null forecaster. Otherwise, people could do as well or better with far less effort than you're putting in. The first 'null forecaster' we reach to is climatology -- predict that things will be the way they 'usually' are. Lately, for sea ice, we've been seeing figures which are wildly different from any earlier observations, so we have to do more to decide what we mean by 'climatology' for sea ice. I noticed that the 50's, 60's, and 70's up to the start of the satellite era had as much or somewhat more ice than the early part of the satellite era (see Chapman and Walsh's data set at the NSIDC). My 'climatological' value for the purpose of making my prediction was 7.38 million km^2, the average of about the first 15 years of the satellite era. A 30 year average including the last 15 years of the pre-satellite era would be about that or a little higher. Again, that figure is part of a distribution, since even before the recent trend, there were years with more or less (than climatology) ice covers.
It may be a surprise, but we also should consider the natural variability in looking at the observed value for the month. Since we're really looking towards climate, we have in mind that if the weather this summer were warmer, there'd be less September ice. And if it were colder, or different wind patterns, there would have been more ice this September. Again, the spread is the 0.47 (at least that's my estimate for the figure).
I'll make the assumption (because otherwise we don't know what to do) that the ranges form a nice bell curve, also known as 'normal distribution', also known as 'Gaussian distribution'. We can then plot each distribution -- from the observed, the prediction, and what climatology might say. They're in the figure:
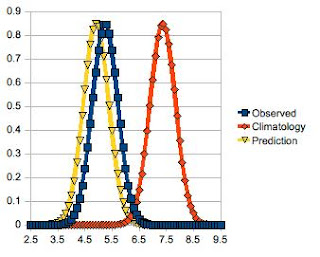
This is one that makes a lot of sense immediately from the graphic. The Observed and Prediction curves overlap each other substantially, while the curves for Observed and Climatology are so far from each other that there's only the tiniest overlap (near 6.4). That tiny overlap occurs for an area where the curves are extremely low -- meaning that neither the observation nor the climatology is likely to produce a value near 6.4, and it gets worse if (as happened) what you saw was 5.25.
The comparison of predictions gets harder if the predictions have different standard deviations. I could, for instance, have decided that although the natural variability was .47, I was not confident about my prediction method, so taken twice as large a variability (for my prediction -- the natural variability for the observation and for the climatology is what it is and not subject to change by me). Obviously, that prediction would be worse than the one I made. Or at least it would be given the observed amount. If we'd really seen 4.25 instead of 5.25, I would have been better off with a less narrow prediction -- the curve would be flatter, but lower. I'll leave that more complicated situation for a later note.
For now, though, we can look at the people who said that the sea ice pack had 'recovered' (which would mean 'got back to climatology') and see that they were horribly wrong. Far more so than any of the serious predictions in the sea ice outlook (June report, I confess I haven't read all of the later reports). The 'sea ice has recovered' folks are as wrong as a prediction of 3.1 million km^2 would have been. Lowest June prediction by far was a 3.2, but the authors noted that it was an 'aggressive' prediction -- they'd skewed everything towards making the model come up with a low number. Their 'moderate' prediction was for a little over 4.7. Shift my yellow triangle curve 0.2 to the left and you have what theirs looks like -- still pretty close.
To go back to my prediction, it was far better than the null forecaster (climatology), so not 'worthless'. Or at least not by that measure. If the variability were small, however, then the curves would have narrow spikes. If the variability were 0.047, ten times smaller than it is, the curves would be near zero once you were more than a couple tenths away from the prediction. Then the distribution for my prediction would show almost no overlap with the observation and its distribution. That would be, if not worthless (at least it was closer than climatology), at least hard to consider having done much good.