In the first what is climate note, I mentioned that climate has something to do with averaging and expectations, rather than precisely what you saw at any given instant. That's good, but it doesn't tell us a lot about how to average. Let us continue with 'global average surface temperature', as that's a popular variable to pick on. We may discover that other variables (rainfall, fraction of rainfall that's from intense storms, ...) need other techniques. But let's start somewhere.
One thing I observed over in the cherry-picking note was that we don't want our conclusions to depend sensitively on particular choices we make in our data (model, whatever) analysis. In the second testing ideas note, I mentioned that the WMO standard averaging period was 30 years, and the IPCC AR4 was using at times 20 years. But ... do those periods lead to one particular conclusion that we wouldn't get if we changed the averaging period? Conversely, maybe the 7 years used in the note I was criticizing there really are more than sufficient to look at climate change (my criticisms stand regardless, but maybe a proper test could be done).
Fluid dynamics (a course I neglected to mention earlier) gives us a nice way of looking at the problem. Over there, we use the 'continuum approximation'. While we know that air is made up of molecules, it isn't a useful way to look at the atmosphere. There are far (far!) too many molecules for us to work on each of them individually. What we do instead is work on a collection of molecules -- a large enough collection. How we define 'large enough' is to consider a variable of interest, say velocity. If we look into the atmosphere at a single molecule, we could see it heading due east at 1000 m/s. But the next could be heading due west at 1001 m/s. If we only took 1 molecule, we see a huge velocity eastward. If we take 2, we see a small westward velocity. What we have to do for the continuum approximation to work is take enough molecules that if we counted a few more, or a bit fewer, the resulting velocity would still be about the same. We don't want, on the other hand, to average up a very large fraction of the atmosphere. Then we'd be averaging out things that we care about, like hurricanes. So, on the 'too small' side, we see the averages jump around because there aren't enough data points. On the 'too large' side, we'd be averaging over processes that we want to study.
Here's a scatter plot of some climate numbers. I'll tell you later exactly where they came from, what the units are, and all that. But for now, let's just look at them with as few preconceptions as possible. One immediate feature we see is that they jump around a lot, a good 0.2 from one point to the next. The total range is from about -0.6 to +0.8, across the 1543 data points. If we're looking for climate in this set, we want to find the 'continuum' degree of averaging -- enough data points that the average holds steady.
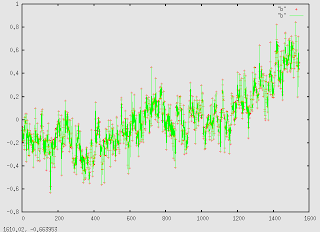
What we observe is weather, what we expect is climate. Our given data point is a weather number. The appropriate average centered on that point is the climate, I'll say. So what I'll compute is the average of a given data point (say the 120th) plus all the data points from 5 before to 5 after that one. And repeat this for 1 before+after to 600 before+after. 600 before and after has us using 1201 data points out of our 1543, so we'll hope that we don't need to use so many. My first attempt is shown here:
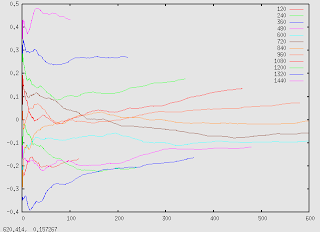
The labels in the upper right are where in the data set I put the center. 120 starts averaging from data point 120 (early in the set) and 1200 is late in the set. They're separated by 120, which means that the averages start including overlapping data when the horizontal axis value is 60 or greater. Still, on this plot we can see that the jumping around that occurs near the start has pretty well settled out by the time we're using plus and minus 300. Now, since that's 40% of the data set (601 points), we're not very surprised. It's enough that to the extent that the curve for 1080 still looks to be rising with an increase in averaging we'd be willing to say that it's a climate change rather than insufficiently long averaging. The curve for 600 looks to have hit a stable value (near -0.1) very quickly, but most seem to be jumping around, including several crossings, in the first ... oh, 240.
So let's draw a new figure, this time only running out to 240 on the horizontal. Also, let's skip the curves for 1440, 1320, 1200, and 360 -- they're not crossing the others (crossing being an interesting feature), they're towards the finish and start of the data record (limiting our ability to extend the curves), they sit at the top and bottom of the figure, meaning that including them gives us less detail to look at the squiggles of the other curves, and they don't seem to do anything interesting that the other curves aren't. Here we go:
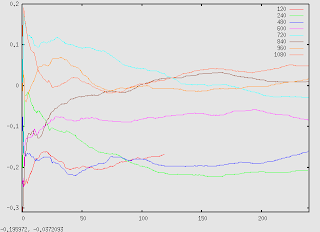
As we look here, we see that different averaging periods give us 'climate' values that vary by 0.1 to 0.2 (depending on which curve we look at). Now that 0.2 was the magnitude of the jitter we saw to start with, so we definitely don't want to do so little averaging that we're still in that range of variation. No point to doing the averaging in the first place, and maybe we'd have to say that there's no such thing as climate.
But if we look some more at the curves, we see that almost all of that variation is, as we'd hoped, near the start (short averaging periods). Between, say, 80 and 240 (end of the plot), the curves vary by only a couple hundredths, a good deal smaller than the 0.2 noise we started with. Hang on to this figure, we'll be coming back to it.
What the data are is the monthly global surface air temperature anomalies, in C, as computed by NCDC. The data start with point 0 being January 1880 and run through July 2008. The 120 data points apart translate to looking at points 10 years apart -- long enough that they shouldn't both be affected by El Nino, and shouldn't be stuck in opposite sides of a solar cycle (5-6 years could give us a problem). In averaging plus and minus 84 (months), we're spanning 14 years centered on the month of current interest.
A 7 year average (total) would be down at 42 (3.5 years either side) on the plot. As you see from the curves, particularly for 840, 960, 1080, once you're that short, your conclusions depend a lot on just what your averaging period is. With no averaging, 840 is about -0.2, 960 is about 0, and 1080 is about +0.2, enormous 'trend' of 0.2 per decade. But if we look out at 42 (3.5 years either side) we see 840 is still coldest, 960 is warmest, and 1080 is in the middle. An up and down with not quite as much down as up. Maybe you'd want to call that a warming trend, but now it's only about 0.025 degrees per decade. Go to 84 (7 years either side) and all three are practically on top of each other, no trend at all. Clearly, if we want to make any sort of stable conclusion about changes, we need more than a few data points. Also clearly, the values have some fuzz left -- the about 0.02 C that they change once we've gotten past about 14 year averaging (84 on the axis).
Back to our initial question about the 30 year (WMO) versus 20 year (IPCC with reluctance) versus 3.5 year averaging (taking a trend out of 7 years lets us only average 3.5 years and gives only 2 data points). The 3.5 years puts us down at 21 months (before and after) of averaging. That's clearly in the zone where conclusions depend strongly on just how long you make you averages, so is cherry-picking zone. The 20 years is 120 months before and after the month of interest. The curves have pretty much settled down at that point. The WMO 30 year period is out at 180 on the curves and things are generally even calmer there. Some of the curving which shows up (see the long version of this figure, above) suggests that 30 years might be too long an averaging period.
Project: I'm putting the data set, my C program, unix script, and my output on my personal website for any who are interested to pull down and see what they get. A different good test is to write it up yourself and see what you get instead. The figures I gave are all for looking at January. Do the conclusions hold the same for July? Other months? What happens if you first average the data year by year? Do I have an error in the program? Do you get the same type of results with other data sets (GISS, HadCRU)?
This is not a rigorous examination, just an exploratory start. Some things have been assumed that shouldn't be for a rigorous version. What are some? The importance of an exploration is not to give us a firm final conclusion, rather to start educating our intuition. When we turn to doing the rigorous analysis, we have some sanity checks at hand.
1 comment:
Hi Robert,
You're being discussed in a detaied coment ove here:
http://scienceblogs.com/illconsidered/2008/09/signal_vs_noise.php
(Just FYI)
Post a Comment